Probabilistic Machine Learning
豆瓣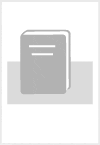
An Introduction
Kevin P Murphy
简介
The second and expanded edition of a comprehensive introduction to machine learning that uses probabilistic models and inference as a unifying approach.
This textbook offers a comprehensive and self-contained introduction to the field of machine learning, including deep learning, viewed through the lens of probabilistic modeling and Bayesian decision theory. This second edition has been substantially expanded and revised, incorporating many recent developments in the field. It has new chapters on linear algebra, optimization, implicit generative models, reinforcement learning, and causality; and other chapters on such topics as variational inference and graphical models have been significantly updated. The software for the book (hosted on github) is now implemented in Python rather than MATLAB, and uses state-of-the-art libraries including as scikit-learn, Tensorflow 2, and JAX.
The book combines breadth and depth. Part 1, on mathematical foundations, covers such topics as probability, statistics, and linear algebra; Part 2, on algorithmic methods, covers such topics as optimization, variational inference, and Monte Carlo sampling; and Part 3, on models, covers such topics as linear models, neural networks, and graphical models. All topics are copiously illustrated with color images and worked examples drawn from application domains including biology, natural language processing, computer vision, and robotics. Exercises are available online. The book is suitable for graduate students and upper-level undergraduates in a variety of quantitative fields, or indeed anyone with an introductory-level college math background.