Hands-On.Gradient.Boosting.with.XGBoost.and.scikit-learn
豆瓣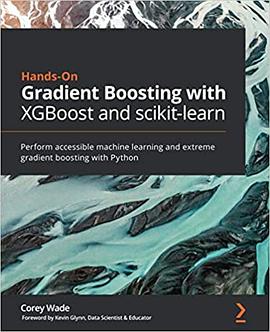
Perform accessible machine learning and extreme gradient boosting with Python
Corey Wade / Kevin Glynn
简介
XGBoost is an industry-proven, open-source software library that provides a gradient boosting framework for scaling billions of data points quickly and efficiently.
The book introduces machine learning and XGBoost in scikit-learn before building up to the theory behind gradient boosting. You'll cover decision trees and analyze bagging in the machine learning context, learning hyperparameters that extend to XGBoost along the way. You'll build gradient boosting models from scratch and extend gradient boosting to big data while recognizing speed limitations using timers. Details in XGBoost are explored with a focus on speed enhancements and deriving parameters mathematically. With the help of detailed case studies, you'll practice building and fine-tuning XGBoost classifiers and regressors using scikit-learn and the original Python API. You'll leverage XGBoost hyperparameters to improve scores, correct missing values, scale imbalanced datasets, and fine-tune alternative base learners. Finally, you'll apply advanced XGBoost techniques like building non-correlated ensembles, stacking models, and preparing models for industry deployment using sparse matrices, customized transformers, and pipelines.
By the end of the book, you'll be able to build high-performing machine learning models using XGBoost with minimal errors and maximum speed.
What you will learn
Build gradient boosting models from scratch
Develop XGBoost regressors and classifiers with accuracy and speed
Analyze variance and bias in terms of fine-tuning XGBoost hyperparameters
Automatically correct missing values and scale imbalanced data
Apply alternative base learners like dart, linear models, and XGBoost random forests
Customize transformers and pipelines to deploy XGBoost models
Build non-correlated ensembles and stack XGBoost models to increase accuracy
contents
Table of Contents
Machine Learning Landscape
Decision Trees in Depth
Bagging with Random Forests
From Gradient Boosting to XGBoost
XGBoost Unveiled
XGBoost Hyperparameters
Discovering Exoplanets with XGBoost
XGBoost Alternative Base Learners
XGBoost Kaggle Masters
XGBoost Model Deployment