The TWIML AI Podcast (formerly This Week in Machine Learning & Artificial Intelligence) - Generative Benchmarking with Kelly Hong - #728
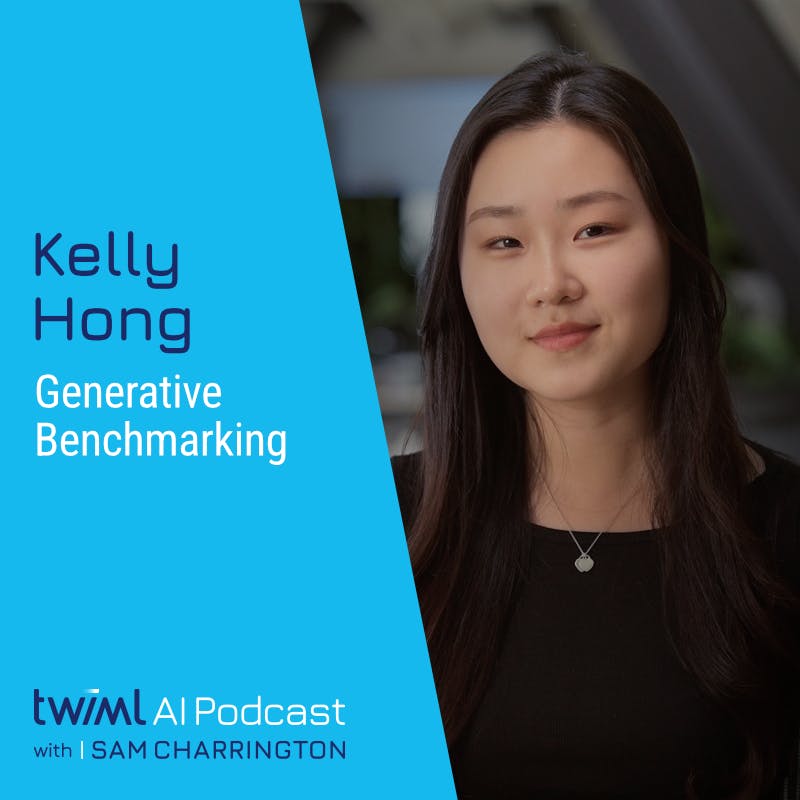
résumé
In this episode, Kelly Hong, a researcher at Chroma, joins us to discuss "Generative Benchmarking," a novel approach to evaluating retrieval systems, like RAG applications, using synthetic data. Kelly explains how traditional benchmarks like MTEB fail to represent real-world query patterns and how embedding models that perform well on public benchmarks often underperform in production. The conversation explores the two-step process of Generative Benchmarking: filtering documents to focus on relevant content and generating queries that mimic actual user behavior. Kelly shares insights from applying this approach to Weights & Biases' technical support bot, revealing how domain-specific evaluation provides more accurate assessments of embedding model performance. We also discuss the importance of aligning LLM judges with human preferences, the impact of chunking strategies on retrieval effectiveness, and how production queries differ from benchmark queries in ambiguity and style. Throughout the episode, Kelly emphasizes the need for systematic evaluation approaches that go beyond "vibe checks" to help developers build more effective RAG applications.
The complete show notes for this episode can be found at https://twimlai.com/go/728.